One of the most important market research buzzwords in 2012 will be big data. Even the future of a large Internet company like Yahoo! can be reduced to this question: What’s your approach on big data (AdAge published an interesting interview with new Yahoo! CEO Scott Thompson about this topic). At first glance, this phenomenon does not appear to be new: There are large masses of data waiting to be analyzed and interpreted. These data oceans have been there before – just think of the huge databases of customer transactions, classic web server log files or astronomical data from the observatories.
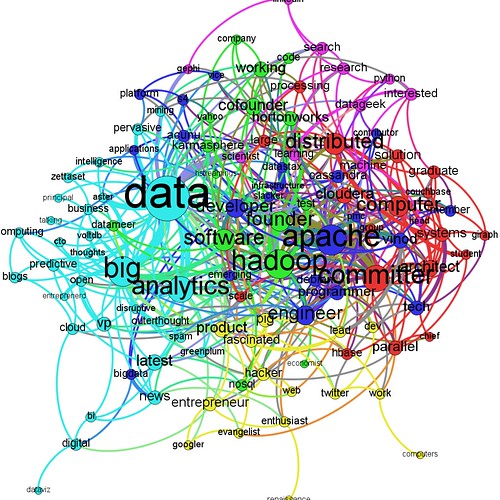
But there does seem to be a new twist on this topic. I believe the following four dimensions really hint at a new understanding of big data:
- Democratization of technology: The tools that are needed to analyze terabytes of data have been democratized. Everyone who has some old desktop PCs in his basement, can transform them into a high-performance Hadoop cluster and start analyzing big data. The software for data gathering, storage, analysis and visualization is more often than not freely available open source software. For those that don’t happen to have a lot of PCs around, there’s always the option of buying computing time and storage at Amazon.
- A new ecosystem: In the meanwhile there is a very active global scene of big data hackers, who are working on various big data technologies and exchanging their use cases in presentations and papers. If you look at the bios of these big data hackers, it becomes apparent that this ecosystem is not dominated by academic research teams, but data scientists working for large Internet companies such as Google, Yahoo!, Twitter or Facebook. This clearly is a difference to e.g. the Python developer community or the R statistics community. In the moment people seem to be moving away from Google, Facebook and the like and joining the ranks of specialized big data companies.
- Network visualization: Visual exploration of data has become almost as important as the classic statistic methodology of looking for causalities. This has the effect that social network analysis (SNA) has gained importance. Almost all social phenomena and large data sets from venture capitalists to LOLcat memes can be visualized and interpreted as networks. Here again, open source software and open data interfaces are playing an important roles. In the near future, software such as the network analysis and visualization tool Gephi can connect directly to the interfaces (APIs) of Facebook, Twitter, Wikipedia and the like and processed the retrieved data immediately.
- New skills and job descriptions: One particular hot buzzword in the big data community is the “data scientist”, who is responsible for gathering and leveraging all data produced in “classic” companies as well as Internet companies. On Smart Planet, I found a very good description of the various new data jobs: There will be a) system administrators who are setting up and maintaining large Hadoop clusters and ensure that the data flow will not be disrupted, b) developers (or “map reducers”) who are developing applications needed to access and evaluate data, c) the data scientists or analysts whose job is telling stories with data and to craft products and solutions and finally d) the data curators who watch over quality and linkage of the data.
To gain a better understanding of how the big data community is seeing itself, I analyzed the Twitter bios of 200 leading big data analysts, developers and entrepreneurs: I transformed all the short bios into a textual network with the words and concepts as nodes and shared mentions of concepts as edges. So, every time, someone is describing himself as “Hadoop committer”, there will be another edge in this network between “Hadoop” and “Committer”. All in all, this network encompasses 800 concepts and 3200 links between concepts. To explore and visualize the network, I reduced it to approximately 15 per cent of its volume and focused on the most frequently mentioned terms (e.g. Big Data, founder, analytics, Apache, Hadoop, Cloudera). The resulting visualization made with Gephi can be seen above.